Bilytica # 1 is one of the top Machine Learning rely on data as the driving force behind every decision and growth. In a digital landscape where speed is crucial, predictive analytics, supported by machine learning, serves as an invaluable tool to organizations in predicting market trends, customer behavior, and operational bottlenecks. With historical data, it enables businesses to make proactive, informed decisions, therefore providing a competitive advantage. This blog discusses the advancement of machine learning within predictive analytics, allowing even meaningless data to become informative business insights that move ahead the business.
Click to Start Whatsapp Chat with Sales
Call #:+923333331225
Email: sales@bilytica.com
Bilytica #1 Machine Learning
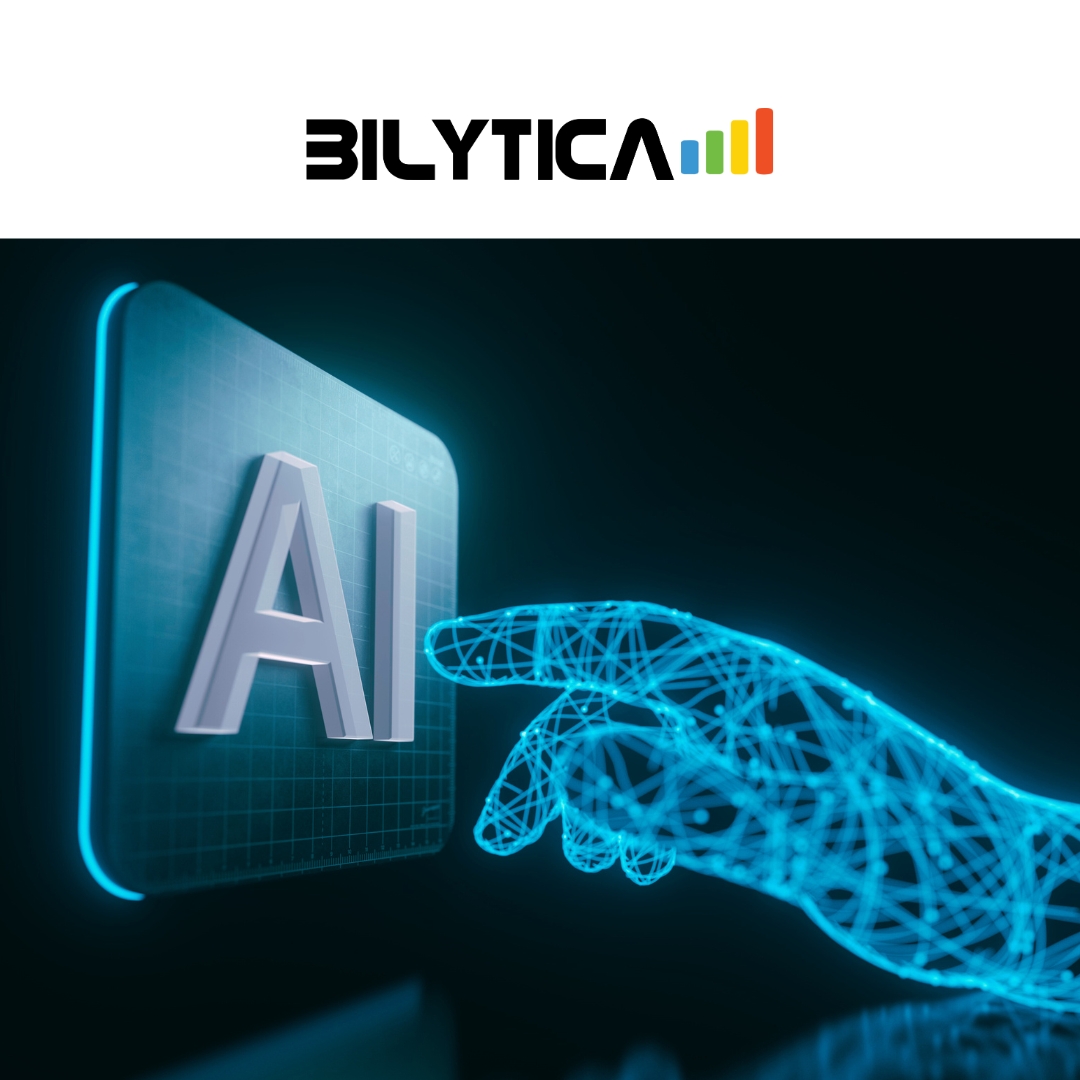
Predictive Analytics in Business – Basics
Predictive analytics refers to the use of statistical algorithms and techniques for data mining combined with Machine Learning algorithms to predict what might happen next. Its applications in this direction have revolutionized industries in finance, retail, health, and manufacturing. Such questions and more can be answered by applying predictive analytics by understanding the patterns and correlation as in which product the consumers might require for the next quarter, what customers will probably churn, and how their supply chain can be avoided in cases of disruption:
The Role of Machine Learning in Predictive Analytics
Machine learning is the backbone of predictive analytics. It allows systems to automatically learn and improve from experience without explicit programming. Here’s how ML fuels predictive analytics for business growth:
It is quicker than traditional analytics tools, thus quickly processing vast and complex data handling. ML algorithms process not only structured data but also unstructured data for different types of diverse information from companies. ML models allow for the detection of sophisticated patterns and trends overlooked by humans. Predictive values are therefore improved. For instance, predicting human preferences or behaviors over several cycles would require laboriously scanning data by the most highly trained data scientists within considerable time.
Continuous Improvement: Machine learning models learn and adapt as they gain more data, always perfecting the accuracy of their predictions. This is a feedback loop that is essential for predictive analytics because it updates real-time predictions to reflect dynamic market movements.
Scalability: Machine learning models scale with the data size; in business, an organization can scale its predictive abilities by gathering more information without increasing the human analysis.
Predictive analytics utilizes several machine learning techniques. Some of the most influential ones are mentioned below.
Regression Analysis: Regression methods, including linear regression, polynomial regression, and decision tree regression, are fundamental for predicting continuous variables, like sales revenue or product price.
Classification Algorithms: The algorithms of logistic regression, SVM, and neural networks classify data points into categories. This is very useful in customer churn prediction, fraud detection, or product recommendation.
Clustering: The clustering algorithms such as K-means or hierarchical clustering group customers or products according to similar characteristics. This helps companies personalize marketing or discover untapped customer segments.
Time-Series Analysis: This helps in prediction based on sequential data, like sales information over time. Algorithms for time-series forecasting include ARIMA, Prophet, and recurrent neural networks (RNN), which help the business predict demand, ensure the right amount of stock, and optimize supply chain.
Customer Retention and Churn Prediction: Predictive analytics with the help of Machine Learning is used to understand which customers are likely to be retained and which will churn. This is important for the business as the churn rate can be used to measure the performance of a company. The models predict the customers who will likely leave engaging with a business so that intervention can occur in good time through certain marketing activities. For instance, telecommunication companies are known to use predictive analytics to target risk-prone customers to retain their services.
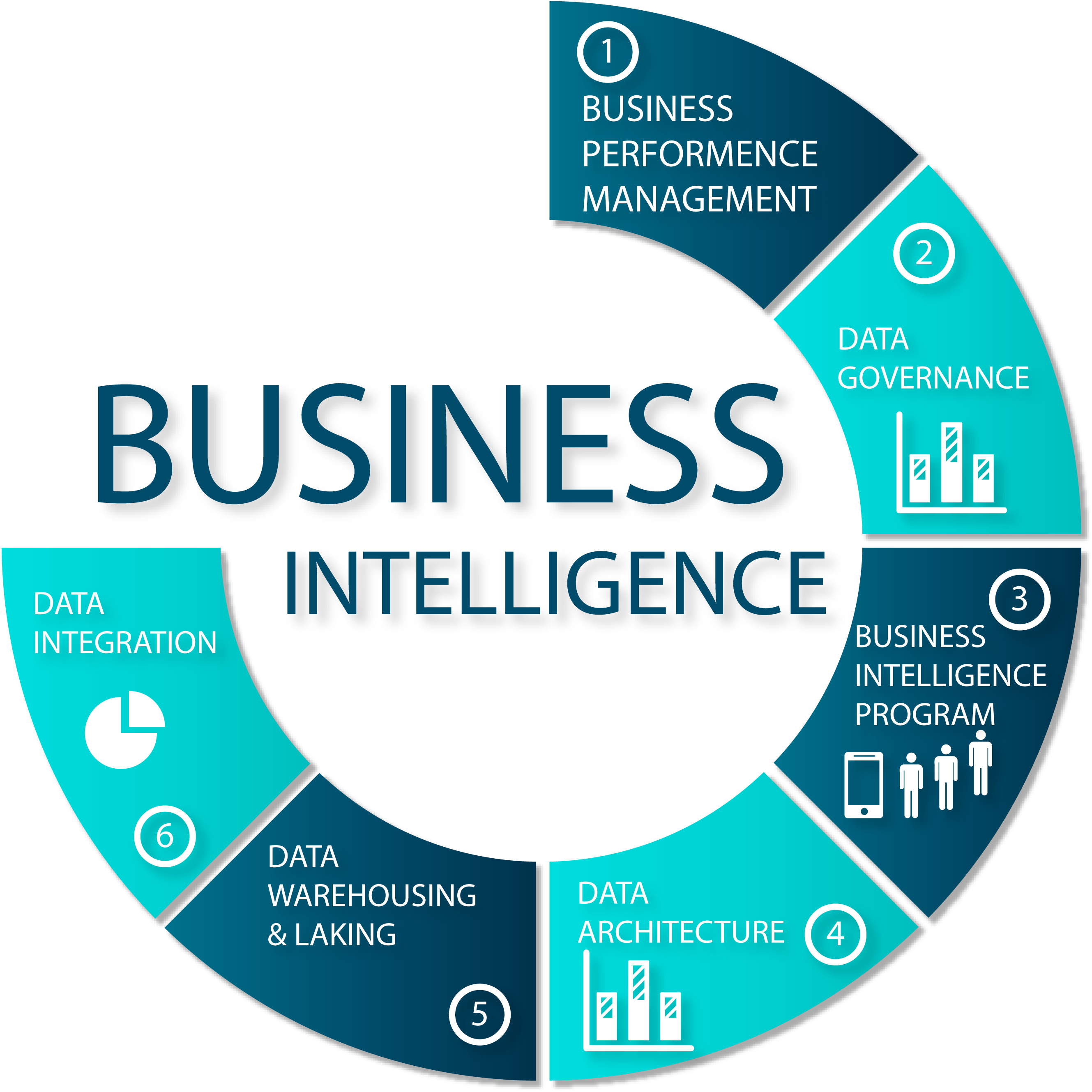
Optimization of Sales and Marketing
With the help of ML-based predictive analytics, analyzing past purchases, customer preferences, and seasonal trends can be optimized. Retailers use predictive models to personalize campaigns by suggesting products in line with the preferences of the customers, which eventually leads to increased conversion rates and customer loyalty.
Supply Chain and Inventory Management
Predictive analytics enables companies to optimize their inventories by ensuring at the right time, appropriate stock will be available in order to satisfy the demand without overstocking. The Data Analysis models predict the demand pattern; thus, companies would modify the supply chain strategy, hence reducing costs and making it more efficient.
Financial Forecasting and Risk Management
It determines credit risk, predicts the stock price, and indicates frauds in finance using predictive analytics. ML algorithms discover signs that signal future risk by doing a pattern analysis of historical financial data to guide business investment decisions.
Human Resources and Employee Retention
Machine learning in HR analytics predicts which employees are most likely to leave so that the company can address these problems before they become significant. Predictive models use such factors as employee engagement, performance, and external market trends to suggest retention strategies.
Challenges of Implementing Predictive Analytics and Machine Learning
Even though machine learning has revolutionized predictive analytics, there are challenges to successful implementation:
Data quality and quantity: High-quality data are needed to train a very precise machine learning model. If the data is imprecise, inconsistent, or inadequate, the function will be affected. Hence organizations would have to pay on data cleaning and preprocessed to rely on data-based good predictions.
Algorithm Selection and Tuning: The selection of appropriate ML algorithm and its tuning for best performance is highly challenging. The model depends on the problem, characteristics of data, and the desired accuracy. Many times, data scientists have to experiment with several models to achieve the desired results.
Scalability and Infrastructure: Training on data is computationally expensive with large datasets for any type of ML. To manage the continuous processes of both training and releasing a new model, an infra of scalable architecture – which must be cloud- centric – is an important ingredient.
Interpretability and Transparency: All those sophisticated deep learning work in closed boxes, while transparency could be a key inhibitor factor in industries bound to rules and regulations strictly.
Ethical and Privacy Concerns: Predictive analytics based on customer data raises ethical and privacy concerns. The businesses need to comply with the data regulations and use the information responsibly concerning the rights of the customers in order to build trust.
Best Practices in the Application of Machine Learning for Predictive Analytics
In using machine learning for predictive analytics, businesses should implement the following best practices to make effective use of the technique:
Invest in Quality Data Infrastructure: A good data foundation is the key. Ensuring that data is accurate, consistent, and well-structured will enhance the performance of ML models.
Start Small, Scale Gradually: Instead of trying to solve all predictive analytics needs at once, start with smaller, manageable projects and scale gradually as the organization gains expertise.
Focus on Model Interpretability: Use interpretable Generative AI wherever possible, especially in industries where interpretability is a concern. SHAP (SHapley Additive exPlanations) can help explain the model’s behavior.
Predictive Insights Should Inform Business Decisions: Predictive analytics is most effective when the insights directly inform business decisions. Encourage data scientists to collaborate with business units to ensure that predictions align with strategic goals.
Responsible Use of Data: Data privacy rules include GDPR and CCPA that require businesses to responsibly use customer data. Businesses need to have strong practices of governance to successfully satisfy these regulations as well as achieve trust among customers.
Conclusion
Predictive analytics has evolved from a theory to a practical tool through machine learning. Through predictive analytics, companies can make data-driven decisions and compete in the fast-changing market by forecasting customer behavior, market trends, and operational challenges.
With the right data, the right algorithms, and proper infrastructure, businesses will unlock the full potential of machine learning and shift from reactive to proactive strategies of fueling sustainable growth. Predictive analytics is not only about technology adoption but also about a forward-looking culture embracing data-driven insight at every decision-making level.
You can explore our other blogs
Generative AI, Power BI , Power Business Intelligence
10-31-2024